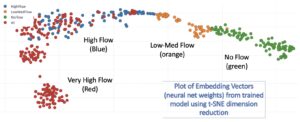
Monitoring and characterization of natural and manmade geologic environments are important aspects of applied geology, and can include monitoring water flow or rock movement, or characterizing the condition of a rock slope or debris flow path. I am currently involved with utilizing neural networks to develop some new monitoring and characterization strategies to help combat the increasing geologic and hydrologic hazards associated with climate change. This is particularly needed in third world countries, where utilizing technologies developed for the mining/petroleum/civil industries is often cost prohibitive. The lightbulb moment for me was listening to a podcast in 2021 given by Pete Warden, who was then the head of Google Brain, a deep learning artificial intelligence research division at Google. He spoke about TinyML, which is the concept of embedding sophisticated neural net routines on very small microcontrollers that are highly energy efficient and can form the basis for field sensors that not only collect data but also analyze, classify, and communicate the results (flood warning for example). This talk will cover the kinds of AI used in TinyML, the potential applications of TinyML to monitor and characterize geologic and hydrologic hazards, and hands-on examples of how easy it is to train a neural net routine and embed it on a small microcontroller. An example we are currently developing is a small sensor that only utilizes sound to characterize water flow in a stream or urban environment, with installations as simple as hanging the sensor from a tree or light pole.